Customer Churn Prediction. Increasing customer retention. Step-by-Step
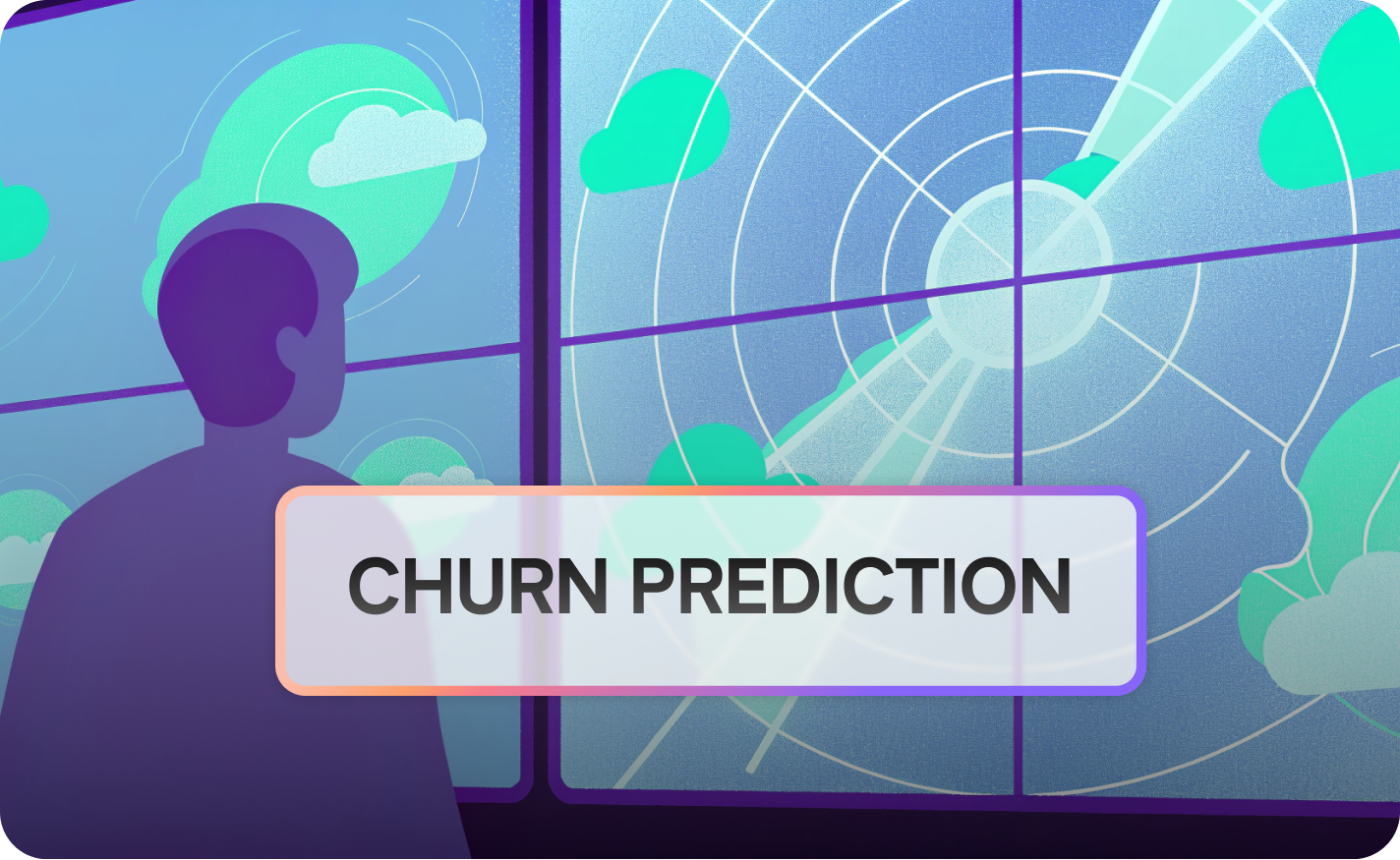
What if you could predict the exact moment a customer decides to abandon your SaaS product?
Imagine peering into the future, spotting the signs of customer churn before it happens, and taking action to keep your customer base intact. In the SaaS industry, where recurring revenue is king, churn prediction isn’t just a fancy buzzword—it’s a strategic necessity.
As Frederick Reichheld of Bain & Company famously stated, "Increasing customer retention rates by 5% increases profits by 25% to 95%." Harvard Business Review: The Value of Keeping the Right Customers Moreover, studies indicate that acquiring a new customer can cost five to seven times more than retaining an existing one, with some sources suggesting it can be as high as 6-7 times more expensive Forbes Business Council: Customer Retention Versus Customer Acquisition SignalMind: Cost of Acquiring New Customers vs. Retaining.
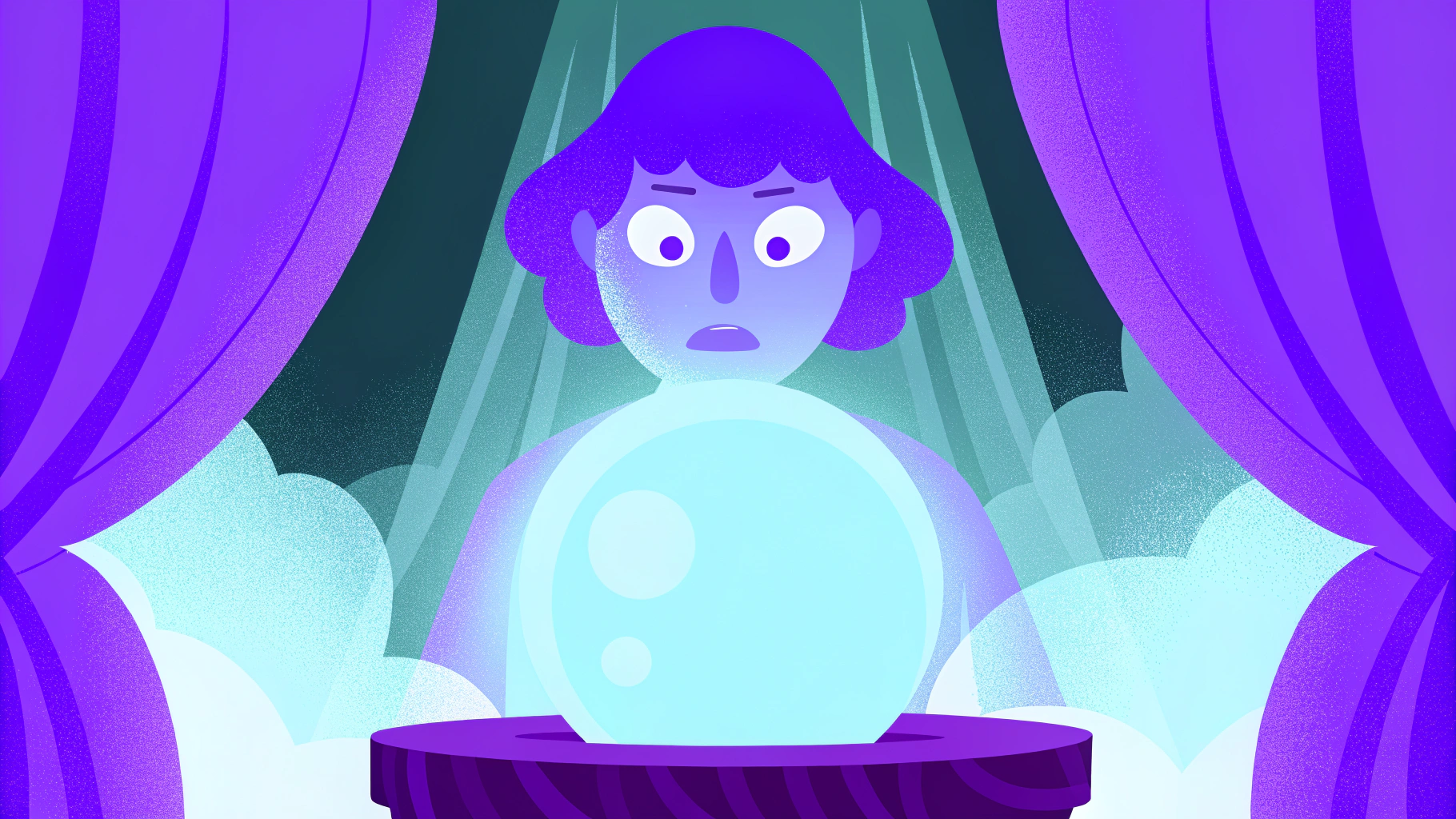
Real-world success stories underscore the impact of churn prediction.
For instance, SaaS startup Groove reduced its churn rate by 71% by identifying key metrics and sending targeted emails to at-risk customers CXL.com: SaaS Churn: 9 Case Studies That Will Help You Make More Money.
Similarly, Mention achieved a 22% churn reduction through segmented support and automated communications CXL.com: SaaS Churn: 9 Case Studies That Will Help You Make More Money. These examples illustrate the tangible benefits of proactive churn management.
Ready to slash churn and boost your bottom line? This guide delivers a technical, actionable roadmap to implement a churn prediction model, featuring tools like LiveSession to turn customer data into retention gold while using data analysis techniques.
Understanding Churn
What’s Churn, Anyway? Let’s start with the basics: what exactly is churn? In SaaS, churn rate measures the percentage of customers who stop using your product or service over a specific period.
Picture this: you begin the month with 1,000 users, and 50 cancel their subscriptions. That’s a 5% churn rate. But there’s more to it. Revenue churn tracks the financial impact:
And then there’s the dream scenario: net negative churn, where revenue from upsells and expansions outpaces losses Lighter Capital: Customer Acquisition vs. Retention: Which Is More Important in SaaS?. Here’s the catch—churn isn’t one-size-fits-all. For some, it’s a canceled subscription; for others, it’s 30 days of inactivity or a downgrade. Define it for your business, because understanding customer behavior is step one to predicting churn.
Importance of Prediction
Why Should You Care About Predicting Churn? Why invest in a crystal ball for your SaaS business? Simple: keeping customers is cheaper than finding new ones—5-25 times cheaper, to be exact Harvard Business Review: The Value of Keeping the Right Customers. Boosting retention by just 5% can lift profits by 25-95%, as demonstrated by research from Bain & Company Harvard Business Review: The Value of Keeping the Right Customers. A predictive model lets you spot churn risk early, intervene with retention strategies, and extend customer lifetime value (LTV). Low churn rates are a key indicator of a healthy SaaS business, signaling to investors that the company has a stable and growing customer base, which can be analyzed using data analysis. Want to reduce churn and secure your revenue stream? Let’s dive into the how.

Churn Prediction for SaaS Products: A Step-by-Step Guide
Introduction
Churn prediction is a game-changer for SaaS businesses looking to reduce customer churn and boost customer retention.
Retaining existing customers is 5-25 times cheaper than acquiring new ones (Width.ai), and a 5% increase in retention can lift profits by 25-95%.
By anticipating customer churn early, you can intervene before customers who cancel slip away, making churn prediction models essential for customer success. This guide walks you through building a churn prediction strategy tailored to your SaaS product, leveraging customer data and tools like LiveSession.
Step 1: Define Churn for Your Product
Defining customer churn is the foundation of your churn prediction strategy. What does churn look like for your SaaS product or service? For some, it’s a subscription cancellation—clean and simple. For others, it’s trickier. Imagine a project management tool: if a user hasn’t logged in for 45 days, are they still a customer?
Maybe, maybe not. It depends on your customer behavior.
Here’s the deal: churn isn’t one-size-fits-all. For a usage-based SaaS, like an analytics platform, customer churn might mean no data uploads for 30 days—clear signs of churn.
For a subscription-based app, it’s that dreaded “Cancel Account” click. Your job is to define it based on product usage data and how customers interact with your service. This clarity ensures your churn prediction model targets those likely to churn.
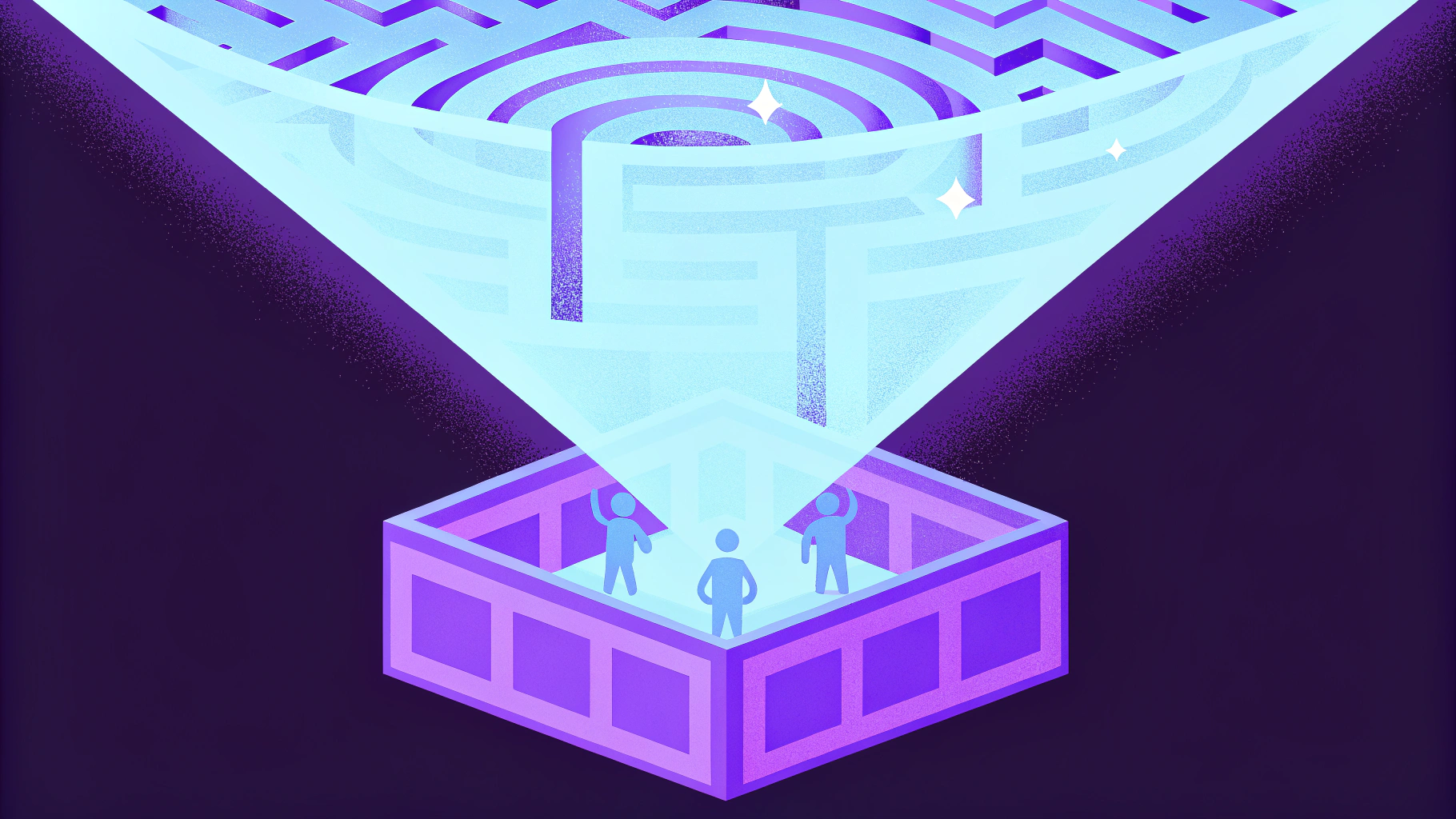
Step 2: Spot the Churn Signals
Now that you’ve defined churn, what’s next in your churn prediction process? Identifying red flags—key customer churn indicators. What do customers do—or stop doing—before they leave? Think fewer logins, downgraded plans, or negative feedback. These are the signs of churn your data analysis needs to uncover.
How do you find them? Dig into historical customer data. Look at customers who cancel in the past. Did they stop using a core feature? Did they send a frustrated support ticket? For example, at a CRM SaaS, we noticed churners often hadn’t logged in for 30 days and had low NPS scores—prime churn risk signals.
Tools like LiveSession shine here. With session recordings, you can track customer behavior in real-time, spotting where users disengage—maybe they’re hitting errors or abandoning features. This data integration enriches your dataset, making your churn prediction more accurate.
Benefits of LiveSession:
- Pinpoint disengagement with real-time data.
- Boost your data points with behavioral insights.
- Sign up and see how it enhances your customer churn prediction.

Step 3: Gather and Prep Your Data
What fuels a churn prediction model? Customer data—lots of it. You’ll need a dataset that tells the story of your customer base: who churned, who stayed, and why. This step is about data integration and preparing a training dataset to predict customer churn.
What data types should you collect?
- Usage Patterns: Login frequency, feature use, time in-app.
- Support History: Ticket volume, sentiment.
- Billing Info: Plan changes, payment hiccups.
- Customer Attributes: Company size, industry.
Clean it up—remove duplicates, fix missing values, and pick relevant data like login trends or feedback scores. Then, split it: 80% for model training, 20% for testing. LiveSession adds depth here with product usage data, like where users struggle, ensuring your data analytics captures the reasons behind customer churn.
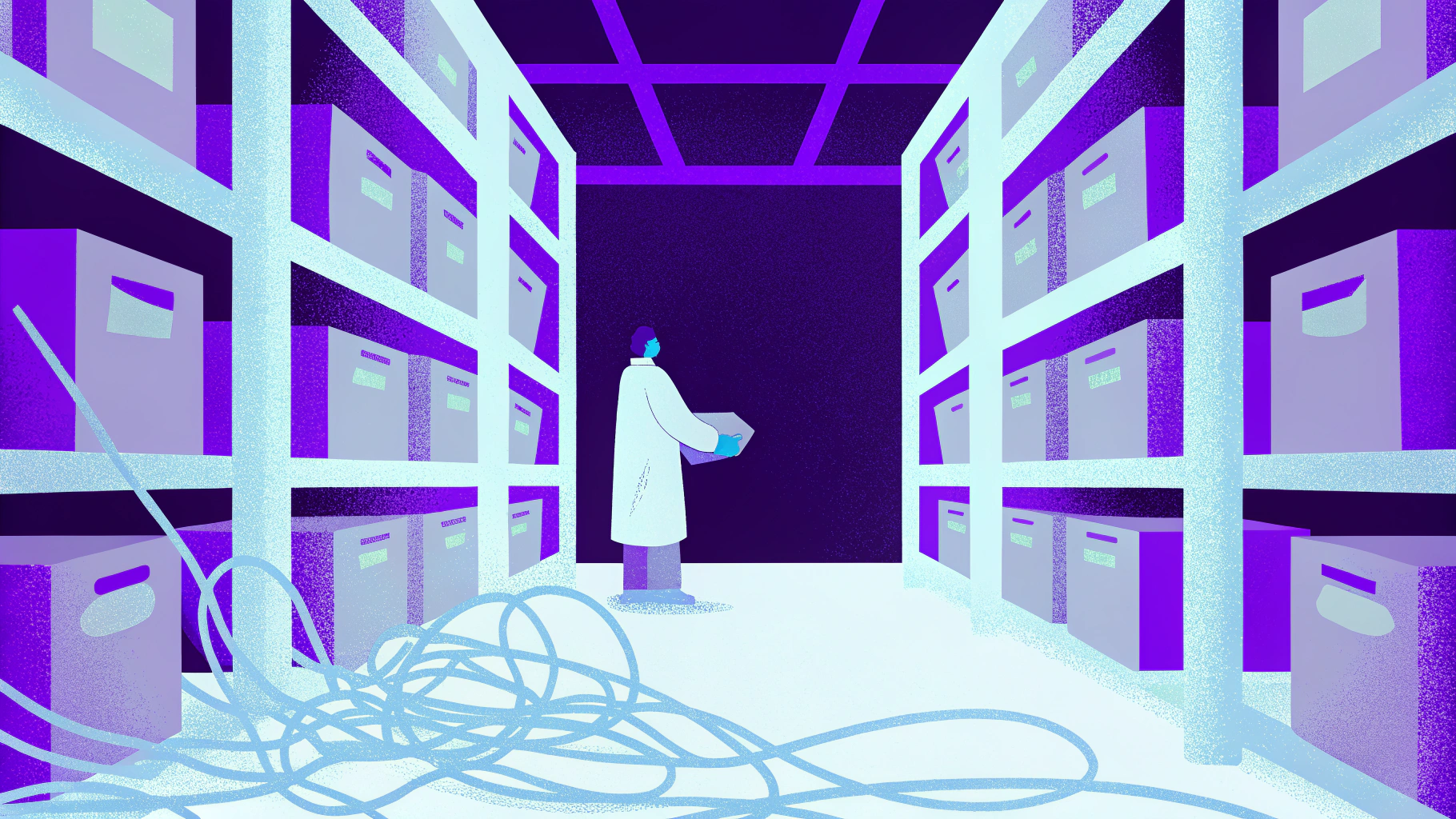
Step 4: Pick Your Prediction Model
With training data ready, it’s time to choose a churn prediction model. Don’t worry if you’re not on a data science team—there’s a machine learning model for everyone.
- Logistic Regression: Simple, interpretable, 70-80% accurate. Great to start predicting churn.
- Decision Trees: Handles complex patterns, 85%+ accuracy.
- Random Forest: A robust machine learning option, 90%+ accuracy for accurate predictions.
Not technical? No-code tools like Akkio or UserMotion use machine learning algorithms to predict churn effortlessly. For advanced users, tools like Power BI can visualize probability of churn. Start simple with logistic regression, then scale to a random forest as your data science grows.
Step 5: Train and Test Your Churn Prediction Model
Here’s where your model training brings the churn prediction model to life. Use that 80% of historical customer data to train it, then test on the 20%. You’re teaching it to predict the likelihood of customer churn.
Key metrics to measure model performance:
- Accuracy: How often is it right?
- Precision: When it flags churn, is it correct?
- Recall: Does it catch most churners? (Key for customer retention.)
- ROC AUC: Overall reliability—aim for above 0.8.
Focus on recall to catch everyone likely to churn. Exploratory data analysis can refine your model, ensuring it uses data to forecast churn effectively.
Step 6: Put Machine Learning to Work
Your model is trained—now implement it. Run it on your customer segments to generate churn risk scores. Think of it as a predictive churn tool: a score from 0 to 1, where 1 means “they’re out the door.”
Set a threshold—say, 0.7—and act. Push scores into your CRM with data integration for real-time data tracking. A customer hits 0.75? That’s your cue to intervene with customer experience strategies to boost customer retention.
Step 7: Act on the Predictions
Predict customer churn without action? Pointless. Use your churn prediction strategy to retain those at higher churn risk.
Retention Strategies:
- Discounts: Offer deals to price-sensitive users.
- Support: Proactively help with onboarding struggles.
- Engagement: Invite them to webinars or share feature tips.
Personalize it. If they’ve stopped using a feature, say, “Hey, we noticed you haven’t tried [X] lately—can we help?” Groove cut churn by 71% with targeted emails, proving customer success drives improving customer retention.
Step 8: Keep your Churn Prediction Strategy Sharp
Don’t let your machine-learning model stagnate. Customer behavior shifts—new features, new markets—so retrain it every 3-6 months with customer feedback and data integration. This keeps your model that predicts churn sharp, reducing churn and boosting customer satisfaction.
LiveSession helps spot new signs of churn. If users ditch a feature, that’s a fresh indicator. Ongoing model training ensures your accurate churn predictions evolve with your diverse customer base.

Conclusion
Building a churn prediction model with machine learning and customer data is your key to customer retention. Follow these steps to predict churn, act on insights, and watch your SaaS thrive. Tools like LiveSession supercharge your efforts with real-time data—sign up to start tackling churn is expensive today.
Overcoming Challenges
Quick heads-up: you’ll hit bumps. Imbalanced data—more stayers than leavers—can skew the overall customer satisfaction metrics. Oversample churners or boost recall. Behavior shifts? Update your model.
Real-World Wins
Let’s talk proof. Mention, a media monitoring SaaS, slashed churn by 22% in a month with customer churn prediction. How? Targeted support and webinars. HubSpot retained 33% of unhappy users with personalized reviews. These aren’t flukes—they’re results of their churn prediction strategy.
Key Takeaway: Predictions + action = retention. It’s that simple.
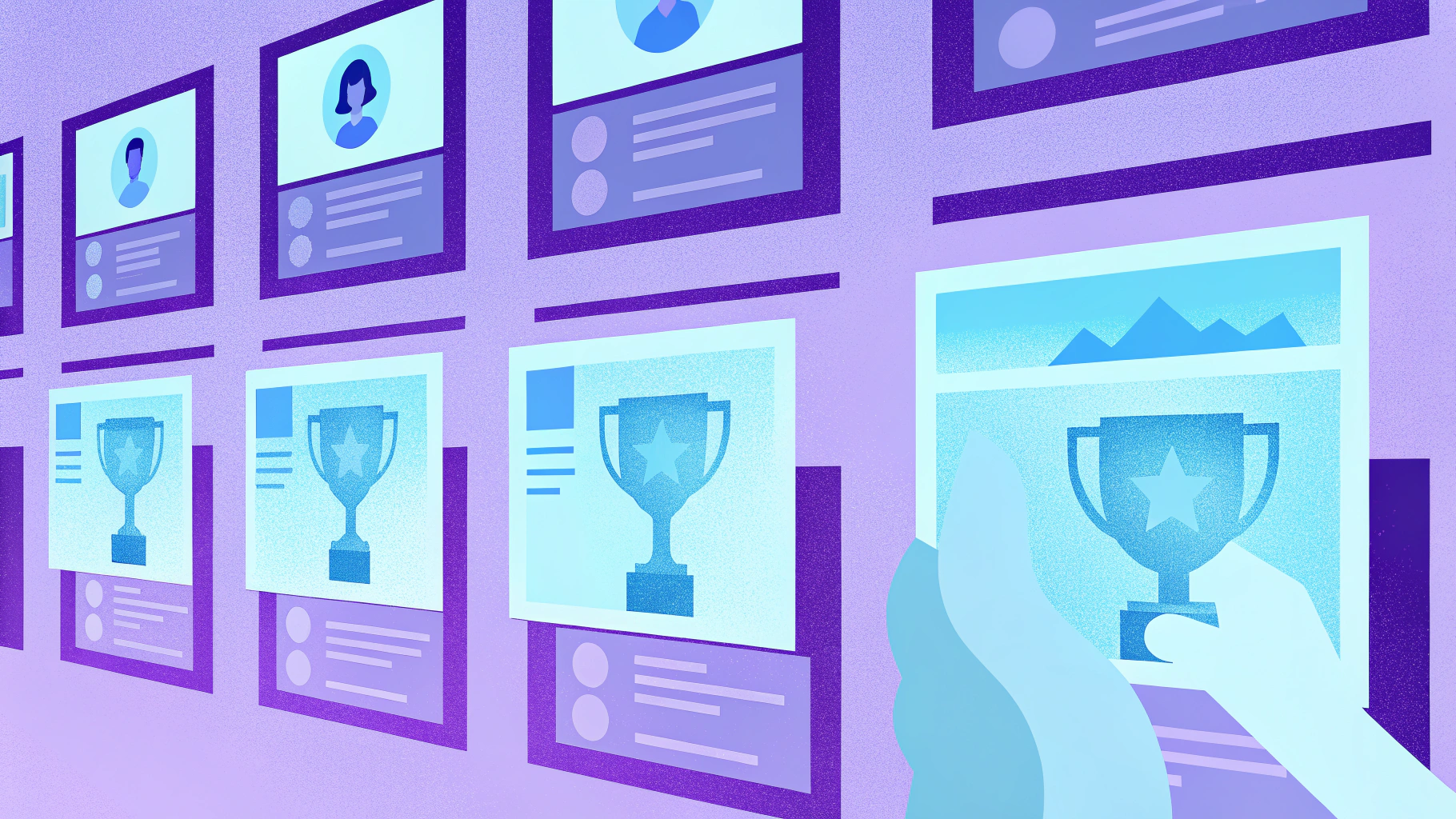
Elevate Customer Retention with LiveSession
One last thing: want sharper predictions? LiveSession is your secret weapon. It records user sessions, showing you the why behind the data—where they struggle, where they drop off.
Wrap-Up
So, here’s the deal: churn prediction isn’t just a buzzword—it’s your ticket to reduce churn, improve customer success, and grow sustainably. Define churn, spot the signs, and build a churn prediction strategy to mitigate the risk of churn.
Don’t stop here. Check out our full guide on reducing churn rates for more. And if you’re serious about mastering customer retention, try LiveSession now. Let’s keep those customers—and your profits—where they belong
Related articles
Get Started for Free
Join thousands of product people, building products with a sleek combination of qualitative and quantitative data.